DETOXIS
IberLEF 2021
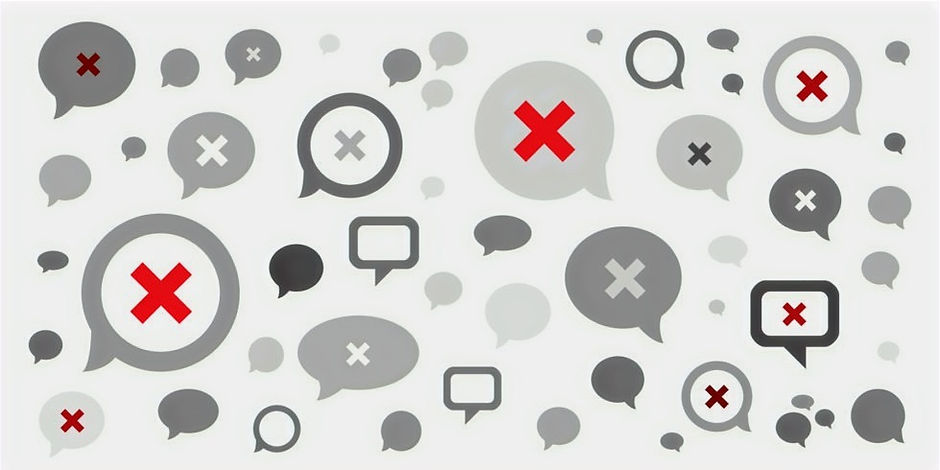
Welcome to DETOXIS at IberLEF 2021
DETOXIS
DEtection of TOXicity in comments In Spanish
The DETOXIS (DEtection of TOxicity in comments In Spanish) task will take place as part of IberLEF 2021, the 3rd Workshop on Iberian Languages Evaluation Forum at the SEPLN 2021 Conference and CEDI 2021, which will be held online on 21 September 2021 in Spain.
(For more information click here)
​
INTRODUCTION
The aim of the DETOXIS task is the detection of toxicity in comments posted in Spanish in response to different online news articles related to immigration. The DETOXIS task is divided into two related classification subtasks: Toxicity detection task and Toxicity level detection task, which are described in section Task.
​
The presence of toxic messages on social media and the need to identify and mitigate them leads to the development of systems for their automatic detection. The automatic detection of toxic language, especially in tweets and comments, is a task that has attracted growing interest from the NLP community in recent years. This interest is reflected in the diversity of the shared tasks that have been organized recently, among which we highlight those held over the last two years: HateEval-2019[1] (Basile et al., 2019) on hate speech against immigrants and women in English and Spanish tweets; TRAC-2 task on Aggression Identification[2] (Kumar et al., 2020) for English, Bengali and Hindi in comments extracted from YouTube; the OffensEval-2020[3] on offensive language identification (Zampieri et al., 2020) in Arabic, Danish, English, Greek and Turkish tweets; GermEval-2019 shared task on the Identification of Offensive Language for German[4] on Twitter (Struẞ et al. 2019); and the Jigsaw Multilingual Toxic Comment Classification Challenge[5], in which the task is focused on building multilingual models (English, French, German, Italian, Portuguese, Russian and Spanish) with English-only training data from Wikipedia comments.
​
DETOXIS is the first task that focuses on the detection of different levels of toxicity in comments posted in response to news articles written in Spanish.
​
The main novelty of the present task is, on the one hand, the methodology applied to the annotation of the dataset that will be used for training and testing the participant models and, on the other hand, the evaluation metrics that will be applied to evaluating the participant models in terms of their system use profile applying four different metrics (F-measure, Rank Biased Precision (Moffat et al. 2008), Closeness Evaluation Measure (Amigó et al., 2020) and Pearson’s correlation coefficient). The methodology proposed aims to reduce the subjectivity of the annotation of toxicity by taking into account the contextual information, i.e. the conversational thread, and by annotating different linguistic features, such as argumentation, constructiveness, stance, target, stereotype, sarcasm, mockery, insult, improper language, aggressiveness and intolerance, which allowed us to discriminate the different levels of toxicity. All this information will be included only in the training dataset that will be used for the task.
​
EXPECTED TARGET COMMUNITY
​The task is open to those participants interested in toxicity and hate speech detection tasks, which is a popular and active area of research due to its impact on modern society. Furthermore, the annotated dataset is a great resource to make an exploratory analysis, as well as to compare the performance of deep learning and classical machine learning models on Spanish toxic expressions rather than on expressions in English, which is the most common language in the majority of public datasets. This task will also attract researchers whose current focus is on data augmentation, pre-training or fine-tuning techniques to achieve state-of-the-art results in tasks lacking many labeled examples.
​
To sum up, since the language of the comments in the dataset is Spanish, the dataset size is prone to data augmentation techniques or transfer learning and the evaluation focus is application-oriented, this proposal will be an attractive choice for beginner, medium and advanced-level NLP scientists to work on.
​
REFERENCES​
Amigó, E., Gonzalo, J., Mizzaro, S., & Carrillo-de-Albornoz, J. (2020). Effectiveness Metrics for Ordinal Classification: Formal Properties and Experimental Results. Proceedings of the 58th Annual Meeting of the Association for Computational Linguistics (ACL 2020).
Basile, V., Bosco, C., Fersini, E., Debora, N., Patti, V., Pardo, F. M. Rangel, Rosso P. & Sanguinetti, M. (2019). Semeval-2019 task 5: Multilingual detection of hate speech against immigrants and women inTwitter. 13th International Workshop on Semantic Evaluation (pp. 54-63), Association for Computational Linguistics.Kumar, R., Ojha, A. K., Malmasi, S., & Zampieri, M. (2018). Benchmarking aggression identification in social media. In Proceedings of the First Workshop on Trolling, Aggression and Cyberbullying (TRAC-2018) (pp. 1-11).
Kumar, R., Ojha, A. K., Malmasi, S., & Zampieri, M. (2020). Evaluating aggression identification in social media. Proceedings of the Second Workshop on Trolling, Aggression and Cyberbullying (pp. 1-5).
Moffat, A., & Zobel, J. (2008). Rank-biased precision for measurement of retrieval effectiveness. ACM Transactions on Information Systems (TOIS), 27(1), 1-27.
Struß, J. M., Siegel, M., Ruppenhofer, J., Wiegand, M., & Klenner, M. (2019). Overview of GermEval Task 2, 2019 shared task on the identification of offensive language. Proceedings of the 15th conference on natural language processing (KONVENS 2019).
Zampieri, M., Nakov, P., Rosenthal, S., Atanasova, P., Karadzhov, G., Mubarak, H., Derczynski, L., Pitenis, Z. & Çöltekin, Ç. (2020). SemEval-2020 task 12: Multilingual offensive language identification in social media (OffensEval 2020). Proceedings of the 14th international workshop on semantic evaluation. arXiv preprint arXiv:2006.07235.
​
-----
[1] https://competitions.codalab.org/competitions/19935
[2] https://sites.google.com/view/trac2/shared-task
[3] https://sites.google.com/site/offensevalsharedtask/
[4] https://projects.fzai.h-da.de/iggsa/the
[5] https://www.kaggle.com/c/jigsaw-multilingual-toxic-comment-classification
​